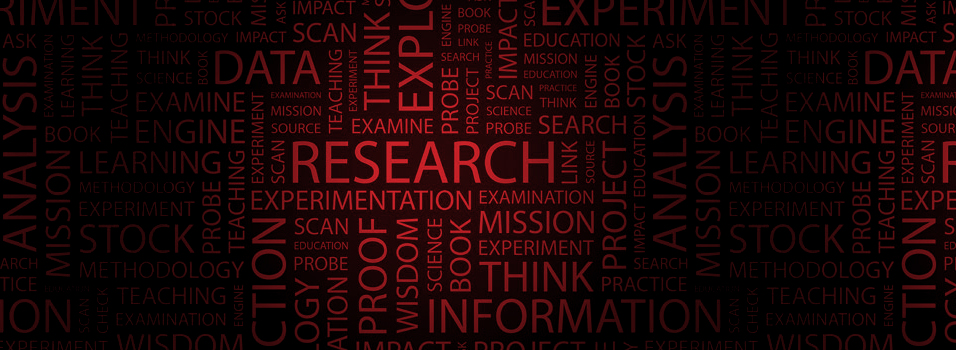
PROCESS CHEMOMETRICS
LABORATORY
@ CERES
RESEARCH
High-dimensional causal-oriented distributed process monitoring
Plant-wide process monitoring is the ultimate integrated application of statistical process monitoring (SPM) to the entire unit, to guarantee the safety of operations, as well as to promote quality, the environment and plant efficiency. Furthermore, and with the digital transformation currently taking place in industry, data became much more abundant and dispersed across the plant.
In this context, conventional methods began to experiment in high-dimensional systems, including the highly successful multivariate approaches, such as Principal Component Analysis, Partial Least Squares, and Independent Component Analysis. These conventional SPM methods are correlation-based and therefore non-causal, which reduces their ability to detect faults by not consider the causal dependencies between variables) and to perform fault diagnosis (due to the well-know “smearing-out” effect). Alternatively, causal SPM methods exploit the process causal network, which maps how process variables relate to each other, to improve process monitoring.
However, all the aforementioned methodologies lose sensitivity in fault detection and diagnosis with the increase of the process dimensionality (the main reason is the high amount of variation introduced from the many multiple sources of information). To overcome this limitation, decentralized monitoring methodologies have been proposed to divide the process variables into several modules, and then perform local monitoring in each module (e.g. hierarchical and multiblock statistical-based monitoring approaches).
APPROACHES ALREADY DEVELOPED INCLUDE:
Network inference and community detection: CNET (Causal Network)
Causality-based decentralized statistical process monitoring schemes: CNET-D (Causal Network - Distributed) & CNET-C (Causal Network - Centralized)