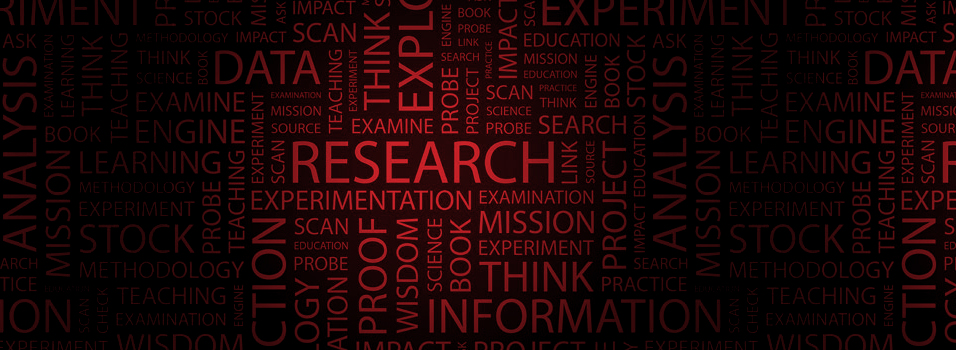
PROCESS CHEMOMETRICS
LABORATORY
@ CERES
RESEARCH
Hybrid Modeling
The chemical processing industry has relied on modeling techniques for process monitoring, control, di- agnosis, optimization, and design, especially since the third industrial revolution and the emergence of Process Systems Engineering. The fourth industrial revolution, connected to massive digitization, made it possible to collect and process large volumes of data triggering the development of data-driven frame- works for knowledge extraction. However, one must not leave behind the successful solutions developed over decades based on first principle mechanistic modeling approaches. At present, both industry and researchers are realizing the need for new ways to incorporate process and phenomenological knowledge in big data and machine learning frameworks, leading to more robust and intelligible artificial intelli- gence solutions, capable of assisting the target stakeholders in their activities and decision processes.
Finding the right structure for a hybrid model is of paramount importance for the achievement of the goal for which a model is destined. The type and quality of information available will play a defining role in the subject. In the classical hybrid modelling liter- ature, the prevalent way to combine the two modeling approaches (mechanistic and data-driven) starts from an analysis of the struc- ture of mechanistic model and its assumptions. A parallel configu- ration can compensate for mechanistic structural mismatch, but if the mechanistic structure is accurate enough, then a serial config- uration is usually a better choice
APPROACHES ALREADY DEVELOPED INCLUDE:
Parallel Hybrid Modeling
Serial Hybrid Modeling
Joint-Y Partial Least Squares