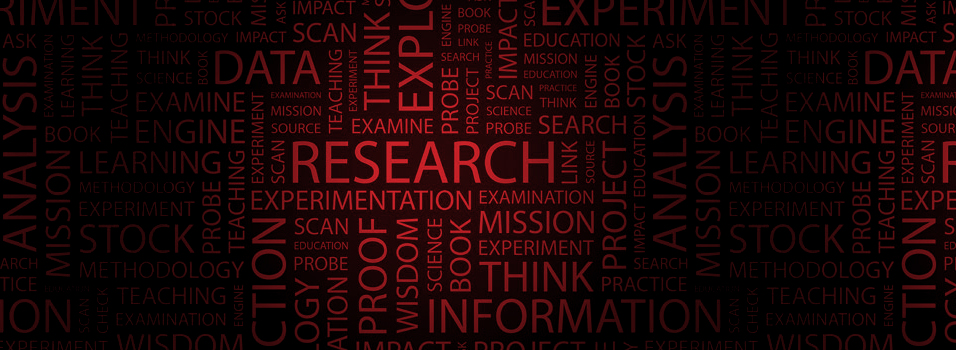
PROCESS CHEMOMETRICS
LABORATORY
@ CERES
Multivariate Image Analysis (MIA)
The automatic acquisition and analysis of images through so called computer and machine vision systems, is already a current reality in a variety of tasks in the Food industry sector, from harvest supervision, product inspection, to process monitoring and control (Brosnan and Sun, 2004). As main drivers, one can point their non-invasive nature, objectivity of the information collected and analyzed, stability of the assessment process, speed and today’s affordability of the necessary hardware components. With the progress in digital cameras and sensing technology, it is now possible to collect images containing a variety of layers of information. The most well-known representatives of such images, are colour images. Available globally since the middle 1980’s, they are the simplest members of the class of spectral images. However, it was the emergence of hyperspectral images (which were initially collected and transmitted by satellites, but now are being used on product and process related applications), multiwavelength X-ray images, among others, that sparked the interest in the development of new tools for their proper processing and analysis. These new images, called in a general sense as multivariate images, carry much more information about the objects and scenes under analysis relatively to the classic ones. However, such potentially useful information is codified in rather complex and high-dimensional data structures, eventually immersed within an also large quantity of irrelevant data. Therefore, they require suitable and effective approaches able to find out and retrieve all the desired relevant information, but that also must be quite efficient and fast, in order to be applied in real world scenarios. In the group several approaches were developed to extract and handle the information contained in images, such as for example:
• Image-based process monitoring and grade assessment of cereal flakes;
• On-line process monitoring of paper surface quality (formation, roughness, waviness);
• Optimal extraction of spectral and spatial features for monitoring random textures;
• Image segmentation using multiscale local features.