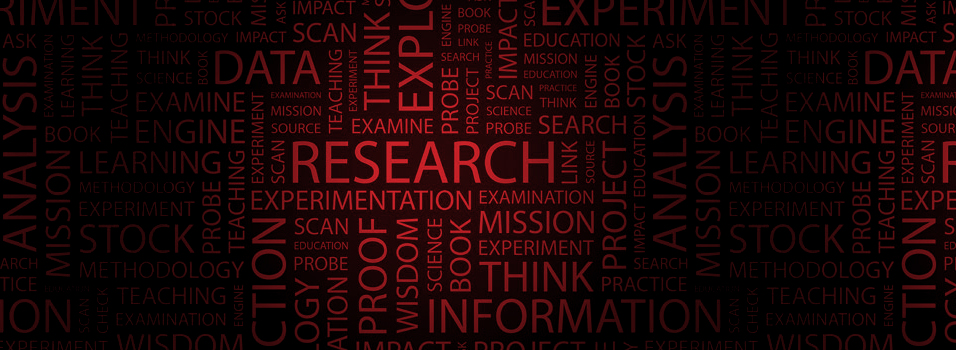
PROCESS CHEMOMETRICS
LABORATORY
@ CERES
RESEARCH
Data Fusion
A wide spectrum of sensor technology is currently available in industrial settings, providing data with different structures and formats. Examples include classical sensors (pressure, temperature, flow, pH, conductivity, etc.); Process Analytical Technology (PAT) (such as Raman spectroscopy, Near-Infrared Spectra (NIR), Nuclear Magnetic Resonance (NMR), chromatography); surface profilometry; grey-level images; color and hyperspectral images; hyphenated instruments; among others. These examples include scalar sensors, as well as first-, second- and high-order tensorial data structures, creating in this way complex Big Data environments.
Taking into account its nature, quite heterogeneous big data environments rise up from the sources amalgam. Data collected also present additional complexity features, such as noise, outliers, sensor drift and degradation, missing values, multirate collection systems, multiresolution aggregation schemes, etc. All these aspects interact in a complex way when trying to extract information from data for a given purpose and need to be properly taken into account. Also, misidentified phenomena can lead to unnecessary costs and economic loss due to lack of quality or wrong estimation. Data fusion arises as an interesting field to explore once it involves methodologies, architectures, models that deal with data streams (of various nature) and combine them in order to compute accurate state estimation of a given entity or system.
APPROACHES ALREADY DEVELOPED INCLUDE:
Bayesian fusion
Regularized Bayesian fusion
Sources classification
Uncertainty estimation
Fusion framework
Mutual error monitoring of sources