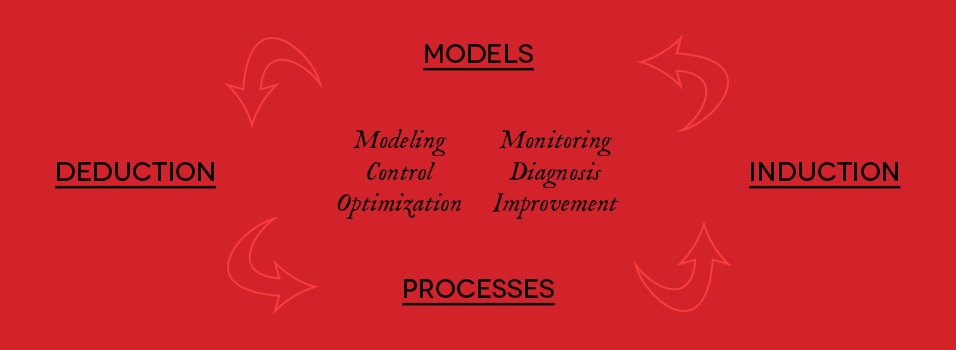
PROCESS CHEMOMETRICS
LABORATORY
@ CERES
The Nature of Industrial Data
Industrial data is the raw material of process chemometrics. It is the mission of process chemometrics to turn this data into value for the organizations. Value involves several dimensions, such as the economical, quality, efficiency, safety and environmental impact. However, data collected from industrial environments present a number of typical features, some of them rising relevant difficulties to the analyst, and that have not be properly addressed so far.
Multivariate structure. Data consists of a number of variables whose values are acquired for the same item, object, time, etc. Therefore, multivariate tools should be employed instead of a battery of univariate methods that overlook eventual interactions between variables.
Dynamic structure. Processes have dynamics and therefore variables will present autocorrelation. This situation is exacerbated by the high acquisition rates of today’s industrial sensors. Methods must be able to cope with this correlation structure along the time axis, besides that between variables.
Multiscale structure. Industrial facilities contain processing units whose operation spans different time-scales. However, most PSE methods are tacitly focused on a single scale of analysis, which necessarily implies a sub-optimal trade-off between the parts of dynamics to be addressed.
Multiresolution structure. Industrial databases contain values corresponding to measurements collected instantaneously, along with others corresponding to averages over different time periods (minutes, hours, days, etc.)
Multirate structure. Measurements may have equal time-resolution (single-resolution) but different acquisition rates.
Asynchronous structure. Measurements collected are not aligned in time, nor are all measurements collected at the same time related to the same product, given the delays involved in industrial units.
Multi-structured. Several data structures are collected simultaneously: scalar measurements (temperatures, flows, pressures), spectra, chromatograms, particle size distributions, grey-level images, multispectral images, hyphenated measurements, etc. These sources must be properly combined/fused.
Noisy. All measurements contain unstructured noise and gross-errors.
Missing data. Databases contain “holes” and blanks, that must be properly addressed during data analysis.
In the PCLab, methods are being developed that simultaneously address an increasing number of these data features in process-related task, such as process/product characterization, modeling, monitoring, control and optimization.