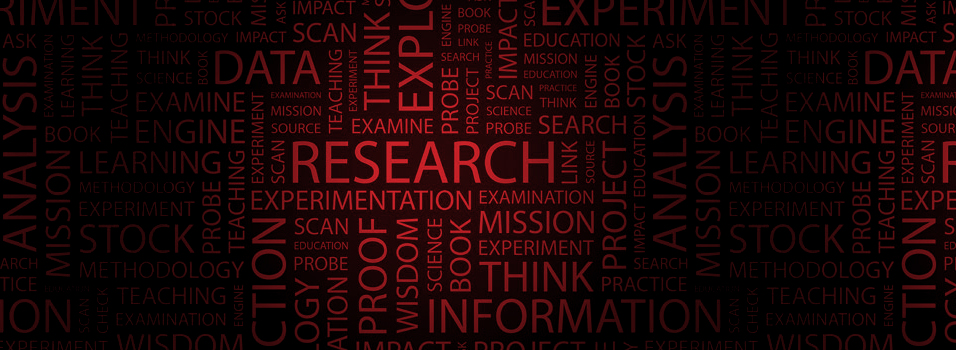
PROCESS CHEMOMETRICS
LABORATORY
@ CERES
DEVELOPMENT
Industrial Soft-Sensors
Soft sensors are software-based procedures for providing estimates of key process variables, in particular those that are hard to measure or only become available after some time delay. They are also known as inferential sensors, and can be developed either strongly relying on a priori knowledge about the processes, using a model-based approach built upon first principles models (FPM), or based on data collected from the process, in which case they are known as data-driven. Model-based soft sensors can provide their estimates for key process variables through a Kalman filtering framework (Jazwinsky, 1970). On the other hand, data-driven soft sensors are implemented using one of the many data-driven predictive tools available, such as principal components regression (PCR) (Jackson, 1991), partial least squares (PLS) (Martens & Naes, 1989), artificial neural networks (ANN) (Bishop, 1995) and support vector machines (SVM) (Vapnik, 2000). At PC Lab we develop mainly data-driven soft sensors, in particular a class of approaches that are able to handle the multiresolution nature of industrial data.
APPROACHES ALREADY DEVELOPED INCLUDE:
Multiscale system identification
Multiscale estimation and Kalman-filtering
A network-induced supervised learning (NI-SL) framework
PAT Soft Sensors (PAT - Process Analytical Technology)
Spectral AutoML - An AutoML Software for Constructing Inferential Models from Spectroscopic Data
MR-iPLS - Multiresolution Interval Partial Least Squares
PAC - Prediction Analytics Comparison framework
SS-DAC - Selecting the Best Modelling Approach and Pre-processing for Spectroscopic Data